Recent publications related to computational audiology
Are there any papers you would like to recommend? Please join our peer recommender challenge!
Below you can find a we have collected so far. Please write a short description with the highlights why you recommend the paper in the
comments on the CAN web page. We will approve the comment and use ChatGPT to compile all comments into a running story including graphics as we did before for the papers published in
Q5.
It is nice to see so many VCCA talks turn into papers and pre-prints. Below publications were found using [(Computational
Audiology) OR ((Machine Learning) AND audiology)] in Google Scholar. Did we miss a publication? Please send your suggestion to resources@xxxxxxxxxxxxxxxxxxxxxxxxxx. If you are going to publish a paper related to computational audiology, consider adding
as key word [Computational Audiology’] to make your paper better findable for your peers and us.
Alohali, Y. A., Abdelsamad, Y., Mesallam, T., Almuhawas, F., Hagr, A., & Fayed, M. S. (2022).
Predicting electrode array impedance after one month from cochlear implantation surgery (arXiv:2205.10021). arXiv.
http://arxiv.org/abs/2205.10021
Alonso-Valerdi, L. M. (2022). Analysis of Electrophysiological Activity of the Nervous System: Towards Neural Engineering Applications. In
Biometry. CRC Press.
Alonso-Valerdi, L. M., Torres-Torres, A. S., Corona-González, C. E., & Ibarra-Zárate, D. I. (2022). Clustering approach based on psychometrics and auditory event-related potentials to evaluate acoustic therapy effects.
Biomedical Signal Processing and Control, 76, 103719.
https://doi.org/10.1016/j.bspc.2022.103719
Bastas, G., Kaliakatsos-Papakostas, M., Paraskevopoulos, G., Kaplanoglou, P., Christantonis, K., Tsioustas, C., Mastrogiannopoulos, D., Panga, D., Fotinea, E., Katsamanis, A., Katsouros, V., Diamantaras, K., & Maragos, P. (2022).
Towards a DHH Accessible Theater: Real-Time Synchronization of Subtitles and Sign Language Videos with ASR and NLP Solutions.
https://doi.org/10.1145/3529190.3534770
Bellandi, V. (2023). A Big Data Infrastructure in Support of Healthy and Independent Living: A Real Case Application. In C. P. Lim, A. Vaidya, Y.-W. Chen, V. Jain, & L. C. Jain (Eds.),
Artificial Intelligence and Machine Learning for Healthcare: Vol. 2: Emerging Methodologies and Trends (pp. 95–134). Springer International Publishing.
https://doi.org/10.1007/978-3-031-11170-9_5
Borole, Y. D., & Raut, R. (2022). Machine‐Learning Techniques for Deaf People.
Machine Learning Algorithms for Signal and Image Processing, 201–217.
Cantu, M. A., & Hohmann, V. (2022). Enhancement of Hearing Aid Processing Via Spatial Spectro-Temporal Post-Filtering with a Prototype Eyeglass-Integrated Array.
2022 International Workshop on Acoustic Signal Enhancement (IWAENC), 1–5.
https://doi.org/10.1109/IWAENC53105.2022.9914762
Casolani, C., Harte, J. M., & Epp, B. (2022). Categorization of tinnitus listeners with a focus on cochlear synaptopathy.
PLOS ONE, 17(12), e0277023.
https://doi.org/10.1371/journal.pone.0277023
Chan, J., Glenn, A., Itani, M., Mancl, L. R., Gallagher, E., Bly, R., Patel, S., & Gollakota, S. (2022).
Wireless earbuds for low-cost hearing screening (arXiv:2212.05435). arXiv.
http://arxiv.org/abs/2212.05435
Diehl, P. U., Singer, Y., Zilly, H., Schönfeld, U., Meyer-Rachner, P., Berry, M., Sprekeler, H., Sprengel, E., Pudszuhn, A., & Hofmann, V. M. (2022).
Restoring speech intelligibility for hearing aid users with deep learning (arXiv:2206.11567). arXiv.
http://arxiv.org/abs/2206.11567
Drakopoulos, F., & Verhulst, S. (2022). A Differentiable Optimisation Framework for The Design of Individualised DNN-based Hearing-Aid Strategies.
ICASSP 2022 - 2022 IEEE International Conference on Acoustics, Speech and Signal Processing (ICASSP), 351–355.
https://doi.org/10.1109/ICASSP43922.2022.9747683
EBSCOhost | 156790805 |
Auditory Evoked Potential-Based Hearing Loss Level Recognition Using Fully Convolutional Neural Networks. (n.d.). Retrieved October 18, 2022.
Fawcett, T. J., Longenecker, R. J., Brunelle, D. L., Berger, J. I., Wallace, M. N., Galazyuk, A. V., Rosen, M. J., Salvi, R. J., & Walton, J. P. (2023). Universal automated classification of the acoustic startle reflex using machine learning.
Hearing Research, 428, 108667.
https://doi.org/10.1016/j.heares.2022.108667
Guiraud, P., Moore, A. H., Vos, R. R., Naylor, P. A., & Brookes, M. (2022). Machine Learning for Parameter Estimation in the MBSTOI Binaural Intelligibility Metric.
2022 International Workshop on Acoustic Signal Enhancement (IWAENC), 1–5.
https://doi.org/10.1109/IWAENC53105.2022.9914725
Haglund, A. (n.d.). Artificial Intelligence for Sign Language Recognition and Translation. 47.
Harris, K. C., & Bao, J. (2022). Optimizing non-invasive functional markers for cochlear deafferentation based on electrocochleography and auditory brainstem responses.
The Journal of the Acoustical Society of America, 151(4), 2802–2808.
https://doi.org/10.1121/10.0010317
Investigations
on the Deep Learning Based Speech Enhancement Algorithms for Hearing-Impaired Population—ProQuest. (n.d.). Retrieved October 18, 2022.
Jeng, F.-C., & Jeng, Y.-S. (2022). Implementation of Machine Learning on Human Frequency-Following Responses: A Tutorial.
Seminars in Hearing, 43(3), 251–274.
https://doi.org/10.1055/s-0042-1756219
Jeng, F.-C., Lin, T.-H., Hart, B. N., Montgomery-Reagan, K., & McDonald, K. (2022). Non-negative matrix factorization improves the efficiency of recording frequency-following responses in normal-hearing adults and neonates.
International Journal of Audiology, 0(0), 1–11.
https://doi.org/10.1080/14992027.2022.2071345
Karbasi, M., & Kolossa, D. (2022). ASR-based speech intelligibility prediction: A review.
Hearing Research, 108606.
https://doi.org/10.1016/j.heares.2022.108606
Kassjański, M., Kulawiak, M., & Przewoźny, T. (2022). Development of an AI-based audiogram classification method for patient referral.
2022 17th Conference on Computer Science and Intelligence Systems (FedCSIS), 163–168.
https://doi.org/10.15439/2022F66
Law, B. M. (2022). Reimagining the Hearing Aid in an OTC Marketplace. Leader Live.
https://leader.pubs.asha.org/do/10.1044/leader.FTR1.27112022.aud-otcs-future.32/full/
Lenatti, M., Moreno, -Sánchez Pedro A., Polo, E. M., Mollura, M., Barbieri, R., & Paglialonga, A. (2022). Evaluation of Machine Learning Algorithms and Explainability Techniques to Detect Hearing Loss From a Speech-in-Noise Screening Test.
American Journal of Audiology, 31(3S), 961–979.
https://doi.org/10.1044/2022_AJA-21-00194
Liu, Z., Li, Y., Yao, L., Monaghan, J. J. M., & McAlpine, D. (2022). Disentangled and Side-aware Unsupervised Domain Adaptation for Cross-dataset Subjective Tinnitus Diagnosis (arXiv:2205.03230). arXiv.
http://arxiv.org/abs/2205.03230
López-Caballero, F., Coffman, B., Seebold, D., Teichert, T., & Salisbury, D. F. (n.d.-a). Intensity and inter-stimulus-interval effects on human middle- and long-latency auditory evoked potentials in an unpredictable auditory context.
Psychophysiology, n/a(n/a), e14217.
https://doi.org/10.1111/psyp.14217
Mondol, S. I. M. M. R., Kim, H. J., Kim, K. S., & Lee, S. (2022a). Machine Learning-Based Hearing Aid Fitting Personalization Using Clinical Fitting Data.
Journal of Healthcare Engineering, 2022, e1667672.
https://doi.org/10.1155/2022/1667672
Müller, M., Jiang, Z., Moryossef, A., Rios, A., & Ebling, S. (2022). Considerations for meaningful sign language machine translation based on glosses (arXiv:2211.15464). arXiv.
http://arxiv.org/abs/2211.15464
Neidhardt, A., Schneiderwind, C., & Klein, F. (2022). Perceptual Matching of Room Acoustics for Auditory Augmented Reality in Small Rooms—Literature Review and Theoretical Framework.
Trends in Hearing, 26, 23312165221092920.
https://doi.org/10.1177/23312165221092919
Orduña-Bustamante, F., Padilla-Ortiz, A. L., & Mena, C. (2023). Assessing the benefits of virtual speaker lateralization for binaural speech intelligibility over the Internet.
Applied Acoustics, 202, 109146.
https://doi.org/10.1016/j.apacoust.2022.109146
Paajanen, M. (n.d.). APPLYING MACHINE LEARNING METHODS TO ANALYSE ARTICULATORY DEPENDENCY OF BONE CONDUCTION TRANSFER FUNCTIONS. 58.
Pai, K. V., & Thilagam, P. S. (2022). Hearing Loss Prediction using Machine Learning Approaches: Contributions, Limitations and Issues.
2022 IEEE 3rd Global Conference for Advancement in Technology (GCAT), 1–7.
https://doi.org/10.1109/GCAT55367.2022.9972110
Ramos-de-Miguel, Á., Escobar, J. M., Greiner, D., Benítez, D., Rodríguez, E., Oliver, A., Hernández, M., & Ramos-Macías, Á. (2022). A phenomenological computational model of the evoked action potential fitted to human cochlear implant responses.
PLOS Computational Biology, 18(5), e1010134.
https://doi.org/10.1371/journal.pcbi.1010134
Rennies, J., Röttges, S., Huber, R., Hauth, C. F., & Brand, T. (2022). A joint framework for blind prediction of binaural speech intelligibility and perceived listening effort.
Hearing Research, 108598.
https://doi.org/10.1016/j.heares.2022.108598
Riojas, K. E., Bruns, T. L., Granna, J., Webster, R. J., & Labadie, R. F. (2022). Robotic pullback technique of a precurved cochlear-implant electrode array using real-time impedance sensing feedback.
International Journal of Computer Assisted Radiology and Surgery.
https://doi.org/10.1007/s11548-022-02772-3
Sandström, J., Myburgh, H., Laurent, C., Swanepoel, D. W., & Lundberg, T. (2022). A Machine Learning Approach to Screen for Otitis Media Using Digital Otoscope Images Labelled by an Expert Panel.
Diagnostics, 12(6), Article 6.
https://doi.org/10.3390/diagnostics12061318
Schmitt, M. (n.d.). Bag-of-Words Representations for Computer Audition. 265.
Schröter, H., Escalante-B., A. N., Rosenkranz, T., & Maier, A. (2022). DeepFilterNet2: Towards Real-Time Speech Enhancement on Embedded Devices for Full-Band Audio (arXiv:2205.05474). arXiv.
http://arxiv.org/abs/2205.05474
Schurzig, D., Repp, F., Timm, M. E., Batsoulis, C., Lenarz, T., & Kral, A. (2023). Virtual cochlear implantation for personalized rehabilitation of profound hearing loss.
Hearing Research, 429, 108687.
https://doi.org/10.1016/j.heares.2022.108687
Sivaraman, A., & Kim, M. (2022). Efficient Personalized Speech Enhancement through Self-Supervised Learning.
IEEE Journal of Selected Topics in Signal Processing, 1–15.
https://doi.org/10.1109/JSTSP.2022.3181782
Smith, S. (2022). Translational Applications of Machine Learning in Auditory Electrophysiology.
Seminars in Hearing, 43(3), 240–250.
https://doi.org/10.1055/s-0042-1756166
Souffi, S., Varnet, L., Zaidi, M., Bathellier, B., Huetz, C., & Edeline, J.-M. (2023). Reduction in sound discrimination in noise is related to envelope similarity and not to a decrease in envelope tracking abilities.
The Journal of Physiology, 601(1), 123–149.
https://doi.org/10.1113/JP283526
Svec, A., & Morgan, S. D. (2022). Virtual audiology education tools: A survey of faculty, graduate students, and undergraduate students.
The Journal of the Acoustical Society of America, 151(5), 3234–3238.
https://doi.org/10.1121/10.0010530
Taylor, K., & Sheikh, W. (2022). Automated Hearing Impairment Diagnosis Using Machine Learning.
2022 Intermountain Engineering, Technology and Computing (IETC), 1–6.
https://doi.org/10.1109/IETC54973.2022.9796707
Use
Brain-Like Audio Features to Improve Speech Recognition Performance—ProQuest. (n.d.). Retrieved October 18, 2022.
Wathour, J., Govaerts, P. J., Derue, L., Vanderbemden, S., Huaux, H., Lacroix, E., & Deggouj, N. (n.d.). Prospective Comparison Between Manual and Computer-Assisted (FOX) Cochlear Implant Fitting in Newly Implanted Patients.
Ear and Hearing, 10.1097/AUD.0000000000001314.
https://doi.org/10.1097/AUD.0000000000001314
Wijewickrema, S., Bester, C., Gerard, J.-M., Collins, A., & O’Leary, S. (2022). Automatic analysis of cochlear response using electrocochleography signals during cochlear implant surgery.
PLOS ONE, 17(7), e0269187.
https://doi.org/10.1371/journal.pone.0269187
Zhong, L., Ricketts, T. A., Roberts, R. A., & Picou, E. M. (n.d.). Benefits of Text Supplementation on Sentence Recognition and Subjective Ratings With and Without Facial Cues for Listeners With Normal Hearing.
Ear and Hearing, 10.1097/AUD.0000000000001316.
https://doi.org/10.1097/AUD.0000000000001316
Last edited January 13.
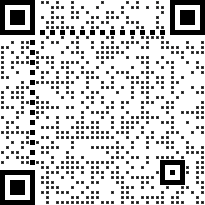
You received this message because you attended VCCA2022, VCCA2021, VCCA2020, or subscribed to the computationalaudiology.com mailings, below are options to opt-out from this mailing list.
|